Congratulations 2023 Pilot Award Recipients!
The Institute of Medical Data Science is excited to announce the recipients of the 2023 Pilot Awards. The pilot program is designed to transform medicine, and the health of the population through innovation leveraging data. This year’s awardees were selected from a diverse group of applicants across informatics, computing, engineering, and medicine. The goal is for funded awards to spark new collaborations between computational science experts and providers.
Pilot funding allows investigators to obtain preliminary data in order to establish a proof of concept and go on to seek larger grants. Investigators supported by the Pilot Program are expanding knowledge relating to enhance clinical breast cancer risk prediction, identification of patients that need Long COVID care, improve prediction and clinical action of extubation failure and developing AI models for tau-Pet images to improve Alzheimer’s disease screening.
Please join us in congratulating our awardees!
“Integration of digital pathology and multiparametric MRI features for improved prediction of patient outcome in triple negative breast cancer“
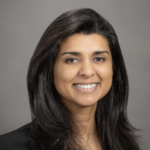
Anum Kazerouni, Phd
Postdoc Scholor - Radiology
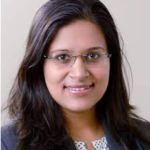
Shachi Mittal, PhD
Assistant Professor - Chemical Engineering
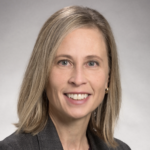
Savannah Partrige, PHD
Professor - Radiology
Towards the goal of precision medicine, our project focuses on the integration of digital pathology and magnetic resonance imaging (MRI) for personalizing treatment of triple negative breast cancer (TNBC). The emerging field of digital pathology employs artificial intelligence (AI) to automate image analysis and provide more comprehensive and quantitative measurement of tissue specimens. Multiparametric MRI can complement findings from pathology samples, providing noninvasive 3D measurement of microenvironment features across a tumor volume. Using retrospective clinical pathology specimens and MRI data in women with TNBC, we are developing novel AI tools to identify pathology signatures from pretreatment biopsies and integrate this information with MRI features in models predictive of TNBC treatment outcome.
“Using machine learning to optimize prediction of extubation failure and clinical action.”
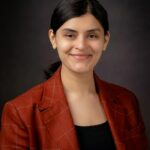
Neha Sathe, MD, MSc
Acting Instructor - Division of Pulmonary, Critical Care, and Sleep Medicine
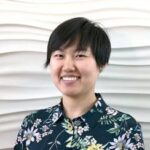
Lucy Lu Wang, PHD
Assistant Professor - Information School Adjunct in BIME, CSE Visiting Scientist, AI2
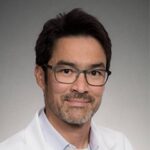
Mark Wurfel, MD, PhD
David J. Pierson Endowed Professor - Division of Pulmonary, Critical Care, and Sleep Medicine
Deciding when and how to extubate patients (i.e. discontinue invasive mechanical ventilation) is a challenge faced by clinicians every day in the intensive care unit. Extubating at the wrong time, or without the right supportive therapies, can increase a patient’s risk for reinitiating mechanical ventilation, and this failure is associated with excess morbidity, mortality, and hospital costs. The overall goal of this project is to develop tools that identify patients at highest risk for extubation failure, bridging expertise in machine-learning, patient safety, and intensive care medicine to optimize the robustness, interpretability, and utility of such tools. The project will yield a machine-learning ready dataset in acute respiratory failure, and has potential to develop predictive tools that reduce clinician workload, support high-stakes decision-making, improve patient outcomes, and reduce hospital costs.
“Interpretable and Multimodal Deep Learning to Enhance Clinical Breast Cancer Risk Prediction.“
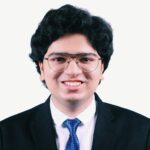
Ojas Ramwala
PhD Student - Biomedical Informatics and Medical Education
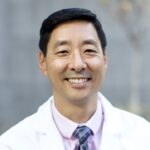
Christoph Lee MD, MS
Professor - Radiology
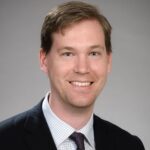
Matt Nyflot, PhD
Associate Professor - Radiation Oncology
Although breast cancer mortality has continued to decline, breast cancer remains the second leading cause of cancer-related death among U.S. women. Moreover, breast cancer risk is not homogeneous across different populations. We aim to develop an explainable deep learning model that integrates imaging and diverse clinical risk factors to predict 5-year breast cancer risk accurately. Using our cloud-based infrastructure, we will rigorously evaluate its performance compared to the state-of-the-art Mirai algorithm and the BCSC clinical risk model. Our work will advance research in interpretable, equitable, and multimodal medical data science.
“Towards Automatic Identification of Patients in Need of Long COVID Care with Natural Language Processing Methods.“
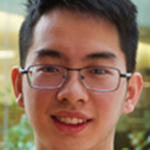
Weipeng Zhou
PhD Candidate - Biomedical Informatics and Medical Education
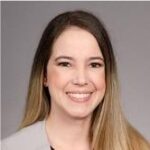
Nikki Gentile, MD, PhD
Assistant Professor - Family Medicine
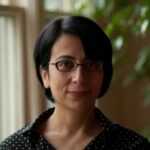
Meliha Yetisgen, PhD
Professor - Biomedical Informatics and Medical Education
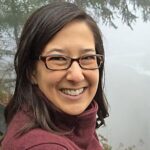
Kari Stephens, PhD
Professor - Family Medicine
This project aims to address the challenges in identifying and managing patients with Long COVID by utilizing natural language processing (NLP) to extract relevant symptoms from electronic health records. Long COVID is a condition characterized by persistent symptoms after COVID-19 illness but lacks a clear definition, which could lead to suboptimal patient care. The project includes three main objectives: (1) the development of an NLP system for automated symptom extraction in clinical notes at UW Medicine; (2) the creation of a comprehensive and validated Long COVID symptom dataset; and (3) an evaluation of Long COVID patient prevalence and characteristics in primary care versus the specialty Long COVID clinic (Post-COVID Rehabilitation and Recovery Clinic). This research aims to enhance healthcare practices by improving the identification and care of Long COVID patients.
“Synthesizing composite tau-PET images from 3D MRI through a novel, diffusion-inspired machine learning framework.“
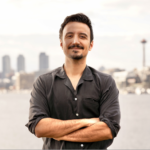
Mehmet Kurt, Phd
Assistant Professor - Mechanical Engineering
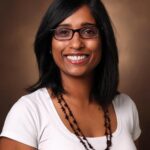
Swati Levendovsky, PhD
Professor - Radiology
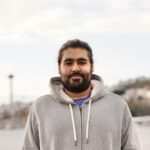
Agamdeep Chopra
PhD Student - Mechanical Engineering
Positron emission tomography (PET) reveals important aspects of Alzheimer’s Disease (AD) pathophysiology including tau protein accumulation, but is not always feasible due to high cost, lack of imaging facilities, and increased risk of radiation exposure. Therefore, this project aims to develop a machine learning framework to predict tau-PET images from 3D magnetic resonance imaging (MRI) as a cost-effective and feasible alternative. Our research intends to pave the way for a clinically meaningful machine learning framework for synthetic PET generation.
Thank You to Our Sponsors
A big thanks to the University of Washington School of Medicine, College of Engineering and School of Public Health for their partnership on this initiative. Additionally, we extend our gratitude to the UW Provost’s Office for their funding support and the eScience Institute for sponsoring cloud computing resources for our pilot teams.
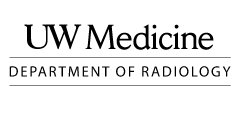
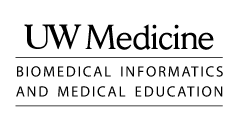
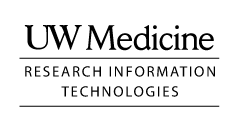
IMDS is supported by the Schools of Medicine and Public Health, the College of Engineering and the Allen School for Computer Science and Engineering