Congratulations 2024 Pilot Award Recipients!
The University of Washington Institute of Medical Data Science is excited to announce the winners of the 2024-25 Pilot Awards. In its second year, this initiative aims to transform medicine and enhance public health through innovative data-driven approaches. Awardees were chosen from a wide range of applicants in fields such as informatics, computing, engineering, and medicine. The goal is for funded awards to spark new collaborations between computational science experts and providers.
Pilot funding for the period of July 1, 2024 to June 30, 2025, enables investigators to obtain preliminary data in order to establish a proof of concept and go on to seek larger grants. Investigators supported by the Pilot Program are working toward improving drug safety surveillance, physician communication of medical errors and and outcomes for older adults suffering from traumatic injuries.
Continue reading to learn more about their projects and join us at the upcoming IMDS Taskforce meeting, where awardees will further detail their approach for discussion.
Please join us in congratulating our awardees!
“Using Temporality, Dose Effect, and Co-Medication to Improve Drug Safety Surveillance.”
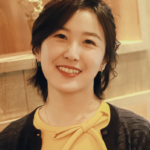
YiFan Wu
PhD Candidate - Biomedical and Health Informatics
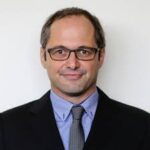
Trevor Cohen MBChB, PhD
Professor - Biomedical and Health Informatics
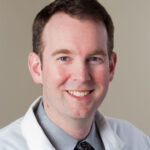
Ian DeBoer, MD
Professor - Medicine, Nephrology
Adverse drug reactions (ADRs) lead to 1.3 million emergency department visits annually, highlighting a major public health issue. Although Electronic Health Record (EHR) data have potential for ADR detection, their effectiveness in post-marketing surveillance is limited by methodological constraints. Previous studies often use cross-sectional EHR data, which fails to adequately account for temporality, outcome definitions, dosage effects, and drug interactions. This research aims to overcome these challenges by employing advanced deep learning models that better represent sequences and interactions, and incorporate dosage information. The focus will be on predicting drug-induced renal disease, leveraging an extensive database of nephrotoxic drugs and laboratory results. This approach aims to enhance ADR detection, improve patient safety, and advance public health outcomes.
“Using Language Models to Rate and Improve the Medical Error Disclosure Communication Skills of Resident Physicians.”
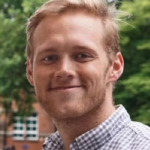
Christopher Rytting, PhD
Postdoctoral Scholar - Allen School of Computer Science & Engineering
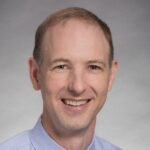
Andrew White, MD
Professor - Medicine
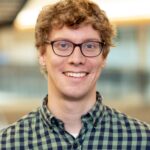
Tim Althoff, PhD
Assistant Professor - Allen School of Computer Science & Engineering
Physicians often fail to provide sufficient information and support after medical errors, leaving patients and families uninformed, distressed and more likely to seek legal action. To address this, UW residencies use the Video-based Communication Assessment (VCA) for practicing communication skills with crowdsourced feedback. Despite its effectiveness, the VCA’s time and cost constraints limit its widespread use. This project aims to develop an algorithm to score VCA responses affordably and quickly. we will develop and evaluate a novel algorithm to score physicians’ error disclosure communication skills from VCA recordings using pretrained language models, retrieval-augmented generation, and supervised fine-tuning. Our goal is to create a system for real-time feedback to learners that has the same reliability and accuracy as crowdsourced human panels of raters.
“Data Pipeline Development for Curation of Geriatric Trauma Outcome prognostication.“
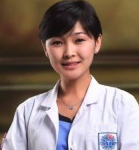
Zoljargal (Zoey) Lkhagvajav
MD, MPH, PhD. Student - Biomedical and Health Informatics
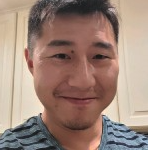
Jim Phuong, PhD
Acting Assistant Professor - Biomedical and Health Informatics
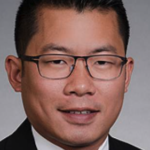
Hamilton Tsang, PhD
Associate Professor, Associate Medical Director of Transfusion Medicine, Laboratory Medicine and Pathology
Traumatic injury is a leading cause of death among older adults in the United States and managing geriatric trauma is highly complex due to diverse data needs and the impact of prior health conditions. Fragmented data storage and sensitive information can hinder the use of data science and AI in this area. At Harborview Medical Center, this project aims to design a data engineering pipeline and data refresh updates for geriatric trauma data research. It includes data quality assessments of the proposed Geriatric Trauma dataset, model application to calculate the Geriatric Trauma Outcomes score, and machine learning to enhance the Geriatric Trauma Outcomes score for real-time clinical prognostication. This initiative would support data engineering automation and improvements to clinical workflows related to geriatric trauma, resuscitation, and blood transfusion.
Thank You to Our Sponsors
A big thanks to the University of Washington School of Medicine, College of Engineering and School of Public Health for their partnership on this initiative. Additionally, we extend our gratitude to the UW Provost’s Office for their funding support and the eScience Institute for sponsoring cloud computing resources for our pilot teams.
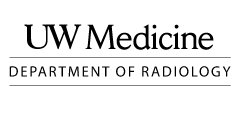
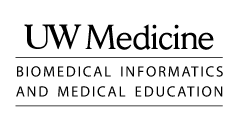
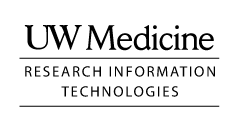
IMDS is supported by the Schools of Medicine and Public Health, the College of Engineering and the Allen School for Computer Science and Engineering